Note
Click here to download the full example code
Investigate a failure from a benchmark¶
The method validate
may raise an exception and
in that case, the class BenchPerfTest
.
The following script shows how to investigate.
from onnxruntime import InferenceSession
from pickle import load
from time import time
import numpy
from numpy.testing import assert_almost_equal
import matplotlib.pyplot as plt
import pandas
from scipy.special import expit
import sklearn
from sklearn.utils._testing import ignore_warnings
from sklearn.linear_model import LogisticRegression
from pymlbenchmark.benchmark import BenchPerf
from pymlbenchmark.external import OnnxRuntimeBenchPerfTestBinaryClassification
Defines the benchmark and runs it¶
class OnnxRuntimeBenchPerfTestBinaryClassification3(
OnnxRuntimeBenchPerfTestBinaryClassification):
"""
Overwrites the class to add a pure python implementation
of the logistic regression.
"""
def fcts(self, dim=None, **kwargs):
def predict_py_predict(X, model=self.skl):
coef = model.coef_
intercept = model.intercept_
pred = numpy.dot(X, coef.T) + intercept
return (pred >= 0).astype(numpy.int32)
def predict_py_predict_proba(X, model=self.skl):
coef = model.coef_
intercept = model.intercept_
pred = numpy.dot(X, coef.T) + intercept
decision_2d = numpy.c_[-pred, pred]
return expit(decision_2d)
res = OnnxRuntimeBenchPerfTestBinaryClassification.fcts(
self, dim=dim, **kwargs)
res.extend([
{'method': 'predict', 'lib': 'py', 'fct': predict_py_predict},
{'method': 'predict_proba', 'lib': 'py',
'fct': predict_py_predict_proba},
])
return res
def validate(self, results, **kwargs):
"""
Raises an exception and locally dump everything we need
to investigate.
"""
# Checks that methods *predict* and *predict_proba* returns
# the same results for both scikit-learn and onnxruntime.
OnnxRuntimeBenchPerfTestBinaryClassification.validate(
self, results, **kwargs)
# Let's dump anything we need for later.
# kwargs contains the input data.
self.dump_error("Just for fun", skl=self.skl,
ort_onnx=self.ort_onnx,
results=results, **kwargs)
raise AssertionError("Just for fun")
@ignore_warnings(category=FutureWarning)
def run_bench(repeat=10, verbose=False):
pbefore = dict(dim=[1, 5], fit_intercept=[True])
pafter = dict(N=[1, 10, 100])
test = lambda dim=None, **opts: (
OnnxRuntimeBenchPerfTestBinaryClassification3(
LogisticRegression, dim=dim, **opts))
bp = BenchPerf(pbefore, pafter, test)
with sklearn.config_context(assume_finite=True):
start = time()
results = list(bp.enumerate_run_benchs(repeat=repeat, verbose=verbose))
end = time()
results_df = pandas.DataFrame(results)
print("Total time = %0.3f sec\n" % (end - start))
return results_df
Runs the benchmark.
try:
run_bench(verbose=True)
except AssertionError as e:
print(e)
0%| | 0/6 [00:00<?, ?it/s]Just for fun
0%| | 0/6 [00:00<?, ?it/s]
Investigation¶
Let’s retrieve what was dumped.
filename = "BENCH-ERROR-OnnxRuntimeBenchPerfTestBinaryClassification3-0.pkl"
try:
with open(filename, "rb") as f:
data = load(f)
good = True
except Exception as e:
print(e)
good = False
if good:
print(list(sorted(data)))
print("msg:", data["msg"])
print(list(sorted(data["data"])))
print(data["data"]['skl'])
['data', 'msg']
msg: Just for fun
['data', 'ort_onnx', 'results', 'skl']
LogisticRegression()
The input data is the following:
if good:
print(data['data']['data'])
[(array([[0.04544796]], dtype=float32),), (array([[0.7556228]], dtype=float32),), (array([[0.7013999]], dtype=float32),), (array([[0.96577233]], dtype=float32),), (array([[0.58974564]], dtype=float32),), (array([[0.7517075]], dtype=float32),), (array([[0.38275772]], dtype=float32),), (array([[0.04419697]], dtype=float32),), (array([[0.16942912]], dtype=float32),), (array([[0.5587462]], dtype=float32),)]
Let’s compare predictions.
if good:
model_skl = data["data"]['skl']
model_onnx = InferenceSession(data["data"]['ort_onnx'].SerializeToString())
input_name = model_onnx.get_inputs()[0].name
def ort_predict_proba(sess, input, input_name):
res = model_onnx.run(None, {input_name: input.astype(numpy.float32)})[1]
return pandas.DataFrame(res).values
if good:
pred_skl = [model_skl.predict_proba(input[0])
for input in data['data']['data']]
pred_onnx = [ort_predict_proba(model_onnx, input[0], input_name)
for input in data['data']['data']]
print(pred_skl)
print(pred_onnx)
[array([[0.49957775, 0.50042225]]), array([[0.50188462, 0.49811538]]), array([[0.50170849, 0.49829151]]), array([[0.50256725, 0.49743275]]), array([[0.5013458, 0.4986542]]), array([[0.5018719, 0.4981281]]), array([[0.50067344, 0.49932656]]), array([[0.49957368, 0.50042632]]), array([[0.49998048, 0.50001952]]), array([[0.50124511, 0.49875489]])]
[array([[0.49957773, 0.50042224]]), array([[0.50188464, 0.49811539]]), array([[0.50170851, 0.49829152]]), array([[0.50256723, 0.49743277]]), array([[0.50134581, 0.49865419]]), array([[0.50187188, 0.49812809]]), array([[0.50067341, 0.49932656]]), array([[0.49957368, 0.50042629]]), array([[0.49998048, 0.50001955]]), array([[0.50124508, 0.49875489]])]
They look the same. Let’s check…
if good:
for a, b in zip(pred_skl, pred_onnx):
assert_almost_equal(a, b)
Computing differences.
if good:
def diff(a, b):
return numpy.max(numpy.abs(a.ravel() - b.ravel()))
diffs = list(sorted(diff(a, b) for a, b in zip(pred_skl, pred_onnx)))
plt.plot(diffs)
plt.title(
"Differences between prediction with\nscikit-learn and onnxruntime"
"\nfor Logistic Regression")
plt.show()
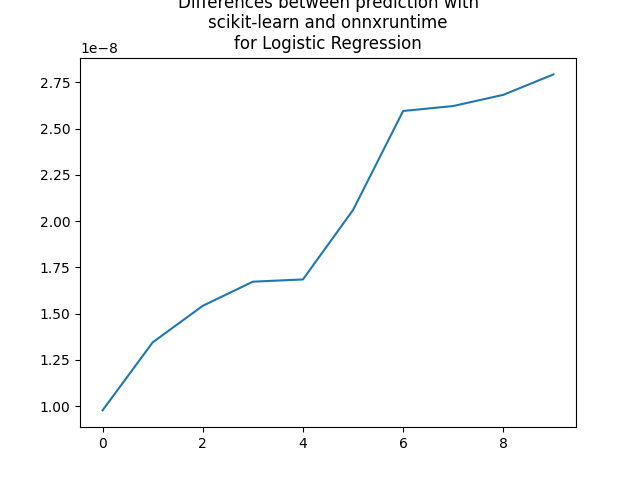
Total running time of the script: ( 0 minutes 1.044 seconds)